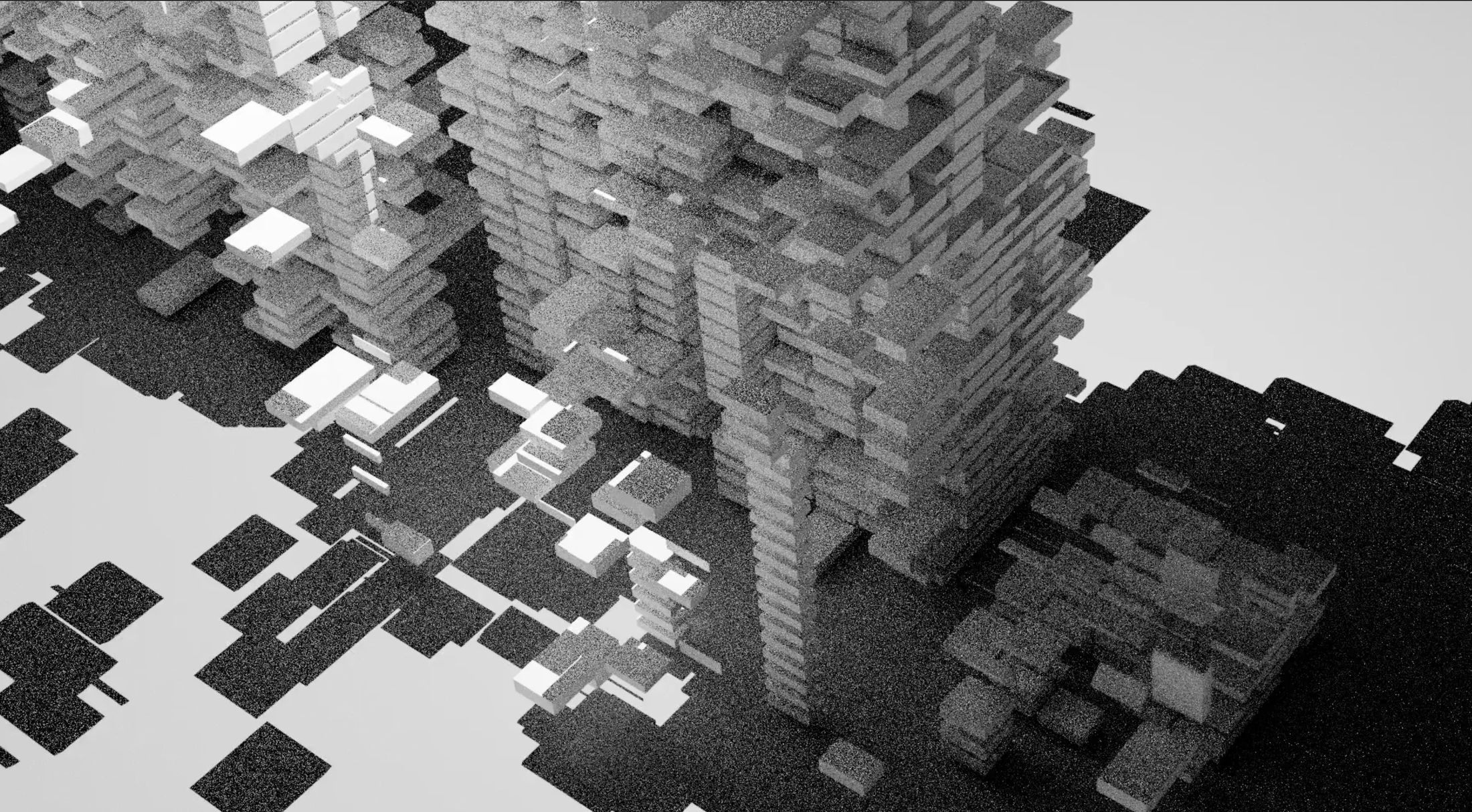
Headless Blender Cycles on Runpod GPUs
Would you like to have 12 RTX 4090s rendering for you in 30 minutes time?
So, I've been working on a Blender project. My first! And I thought I had just finished it, but uploading to YouTube it took all the grain (some of which I had added in post) and turned it into a kind of compression mush. Yes - the carefully constructed linearised workflows - EXR and AgX - now mostly mush.
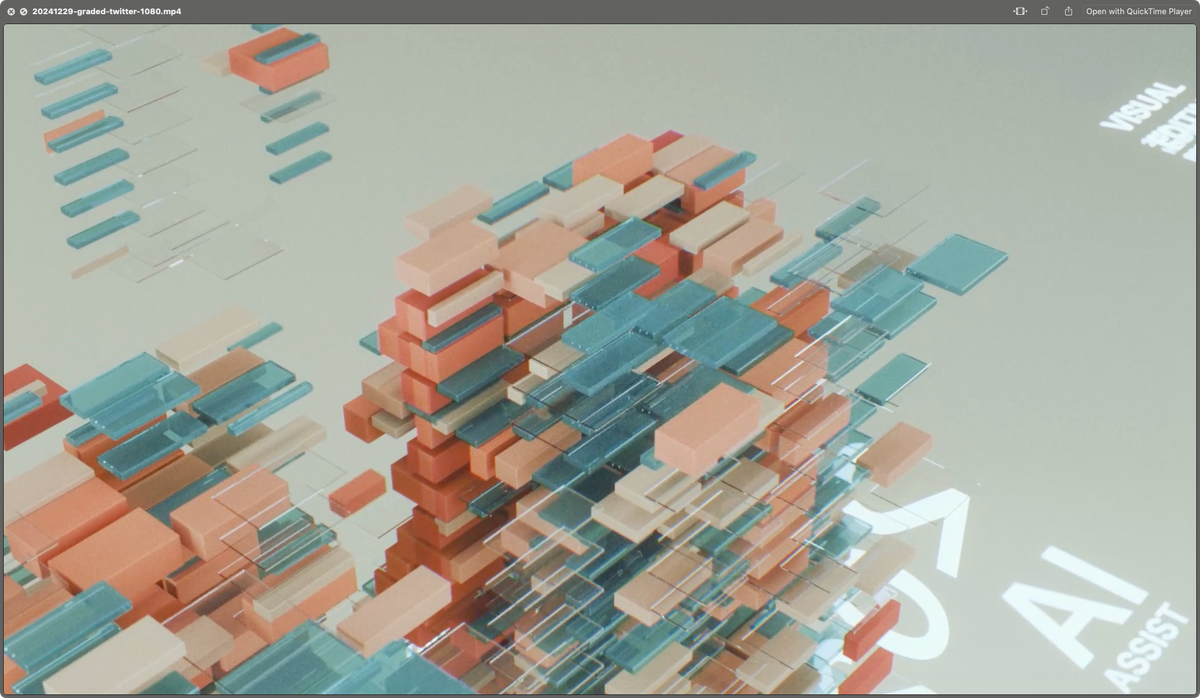
Unsurprisingly perhaps, YouTube doesn't really like grain or noise and doing anything about it demands a bunch of compute. I have around 6k frames and they're taking a week to render on the 3090 I have stuck in a utility closet - with the current settings, which aren't good enough by far. And I would like to go from 1080p to 2K which is 4x the pixels.
So I need more GPUs. More than I can readily buy. Luckily AI suddenly needing a bunch of GPUs makes this something of a common problem these days.
I've used Runpod before to train LoRAs for Mistral and Stable Diffusion and thought I would give it a go. It's a nice service. Paygo from $0 and up. Quick setup.
But it took a lot of time to get this working! Like > 4hrs. Mostly because of really wonky setup artefacts in headless Blender (4.2.0) so I thought I'd tell you, intrepidly rendering reader, how to get this running.
Get a Runpod account. Make some keys for ssh and add them to Runpod. If you aren't used to this kind of stuff - this is the hardest part.
Find a region on Runpod where you can get a bunch of cards attached to one box. I went for 4x 4090s after first looking at the RTX 4000s.
I used this image - look for the highest CUDA version your blender version is foo comfortable with - this worked with 4.2.0:
> RunPod Pytorch 2.4.0runpod/pytorch:2.4.0-py3.11-cuda12.4.1-devel-ubuntu22.04
Give the container 10GB disk and idk 20GB for your persistent disk. Make sure you make the network disk homed to a compute center that supports the flavour compute you want. Their server centres are not created equal. You need to create the disk before you can attach it so you might need to go back and forth a bit.
Blender will want a bunch of packages to run - these install in a few seconds. Also get Blender itself and unpack it.
apt-get update && apt-get install-yxz-utils rsync
apt-get install -y libx11-6 libxi6 libxrender1 libxxf86vm1 libxcursor1 libxrandr2 libglu1-mesa libosmesa6 mesa-common-dev libgl1-mesa-dri libopenal1 libsndfile1 libpython3.10 libboost-locale1.74.0 libegl1 libegl-mesa0 mesa-utils libxkbcommon0 libsm6 libxext6 libxrender1
wget https://download.blender.org/release/Blender4.2/blender-4.2.0-linux-x64.tar.xz
# or whatever version you need
tar xf blender-4.2.0-linux-x64.tar.xz
Now on local, bundle up your scene into a neat directory structure and rsync it over to the server:
rsync -vr -e "ssh -p <runPodPort> -i ~/.ssh/id_rsa" your/scene/location root@<runPodIP>:/workspace/scene
That's basically it. You're kinda of done. I still spent 4 hours on this next step though.
This as you might need to force Blender to use the CUDA units. And you do this with a script. And the order of the params to blender matters. Yeah, when it hits -a it will just start processing. And blender also needs a bit of a break before being able to detect GPUs after startup. A couple of seconds might do nicely. Just you know - hang out for a bit before asking.
I tore out and reinstalled different flavours of CUDA at least 5 times on Runpod today. CUDA is huge and it's time consuming. And Blender wasn't really providing any error messages. So I kept doing the same thing in slightly different ways.
So instead of doing that - where you're going to be running blender - create this file:
import bpy
import time
print("Waiting for device initialization...")
time.sleep(2)
print("Starting CUDA setup...")
try:
bpy.context.preferences.addons["cycles"].preferences.compute_device_type = "CUDA"
print(f"Set compute device type: {bpy.context.preferences.addons['cycles'].preferences.compute_device_type}")
bpy.context.scene.cycles.device = "GPU"
print(f"Set cycles device: {bpy.context.scene.cycles.device}")
bpy.context.preferences.addons["cycles"].preferences.refresh_devices()
time.sleep(1)
devices = bpy.context.preferences.addons["cycles"].preferences.devices
print(f"Available devices: {[d.name for d in devices]}")
if devices:
devices[0].use = True
print(f"Enabled device: {devices[0].name}")
else:
print("No devices found!")
print("CUDA setup completed")
And then invoke Blender with:./blender-4.2.0-linux-x64/blender -b <yourScene.blend> -E CYCLES -P setup_cuda.py -a
See - all the GPUs, so happy now:
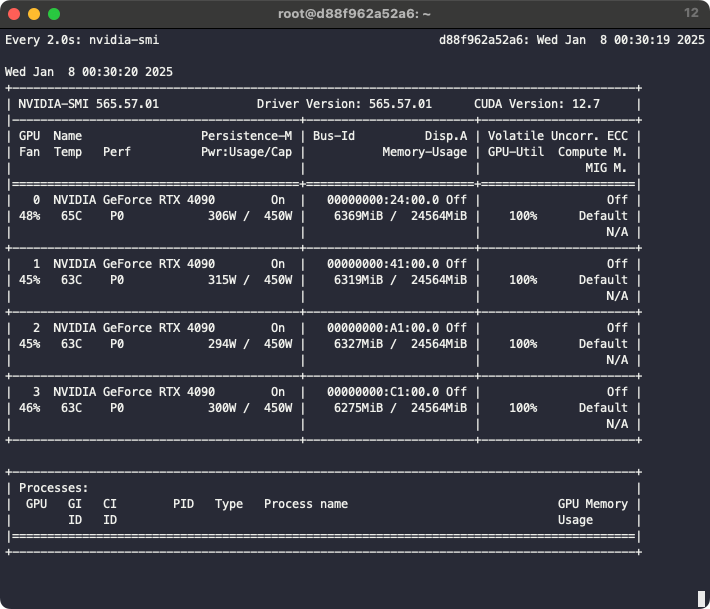
Pro-tip: Blender has startup/shutdown for every frame and doesn't drive the GPUs at 100% even during normal operation so you can eke out another 10-15% perf by running a couple of blenders in each pod if you can fit your scene in the GPU twice.
Also - as the network drive is shared (doh) you can uh - just have lots of these machines rendering to the same volume. Cost scaling is linear so you get to just throw GPUs at your problem - it won't affect the end result: you either run out of money or your problem gets solved. It just happens faster.
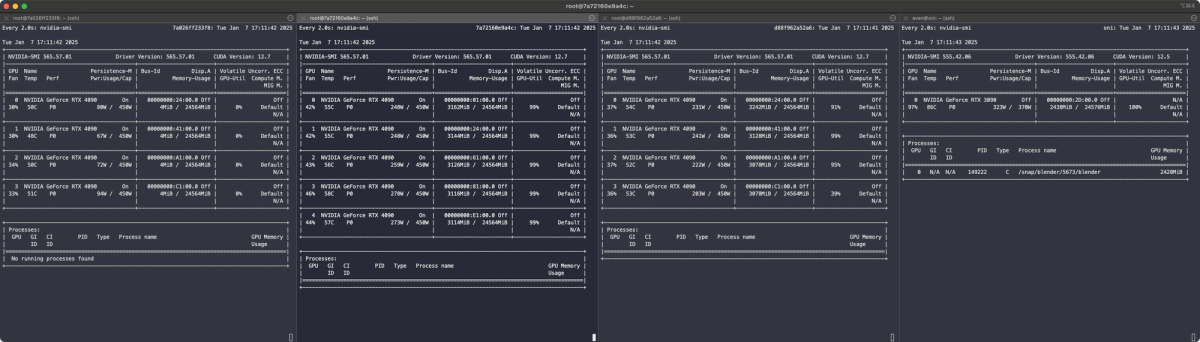
To get your images out of Runpod - either rsync the other way or make an archive in RAM over the wire:ssh -p <RunpodPort> -i ~/.ssh/id_rsa root@<RunpodIP> "cd /workspace && tar czf - frames" > frames_backup.tar.gz
As I'm loathe to miss an opportunity for AI boosterism - the error was actually caught by Claude when looking at this v. inscrutable output I provided:
arrh, we're still getting:
Blender 4.2.0 (hash a51f293548ad built 2024-07-16 06:27:02)
Warning: Falling back to the standard locale ("C")
Read blend: "/workspace/scene/blender/calvis_137_1440p_runpod.blend"
Starting CUDA setup...
Set compute device type to: CUDA
Set cycles device to: GPU
Available devices: []
No devices found!
CUDA setup completed
skipping existing frame "/workspace/frames/0300.exr"
skipping existing frame "/workspace/frames/0301.exr"
skipping existing frame "/workspace/frames/0302.exr"
I0105 20:36:07.527163 5345 device.cpp:36] CUEW initialization succeeded
I0105 20:36:07.528684 5345 device.cpp:38] Found precompiled kernels
and going (I picture it smoking a pipe while saying this):
hmm, interesting. the script runs before blender fully initializes the devices (notice how the CUEW initialization happens after our script output).
I have to admit I would never have spotted this. What even is a CUEW init? Ok, Maybe you aren't AGI yet, and maybe you are just a stochastic parrot, but you still came in clutch buddy.
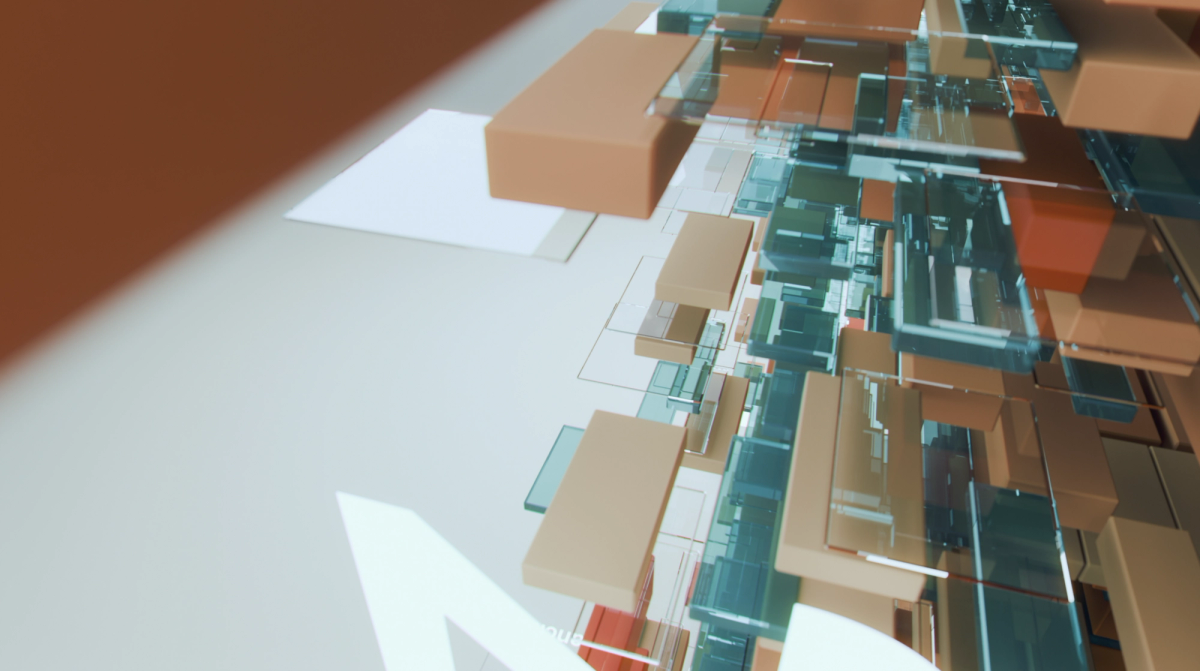